Illustrated Image Analytics
The Illustrated Newspaper Analytics project is developing techniques in computer vision and image processing for large-scale interpretation of historical illustrations. Our source materials are a set of illustrated British newspapers including The Graphic, The Illustrated Police News, and the Penny Illustrated Paper – among the many nineteenth-century periodicals which adapted the technique of wood-engraved illustration to the industrial scale of newspaper publishing. Computational techniques may afford new ways of accessing and analyzing this massive visual archive. This project suggests how image processing techniques can reveal large-scale patterns in the visual language of the nineteenth century, opening further research questions about historical visual culture and graphic knowledge.
Using figure extraction, image matching, segmentation, and other algorithmic techniques, the Illustrated Newspaper Analytics project investigates historical questions and engineers new applications for computer vision using the challenges of humanities data. This research represents an ongoing interdisciplinary collaboration between researchers the NC State Department of English, the NCSU Libraries, and the Laboratory for Analytic Sciences. The 2016 British Library Labs Awards recognized the project as runner-up in the Research category.
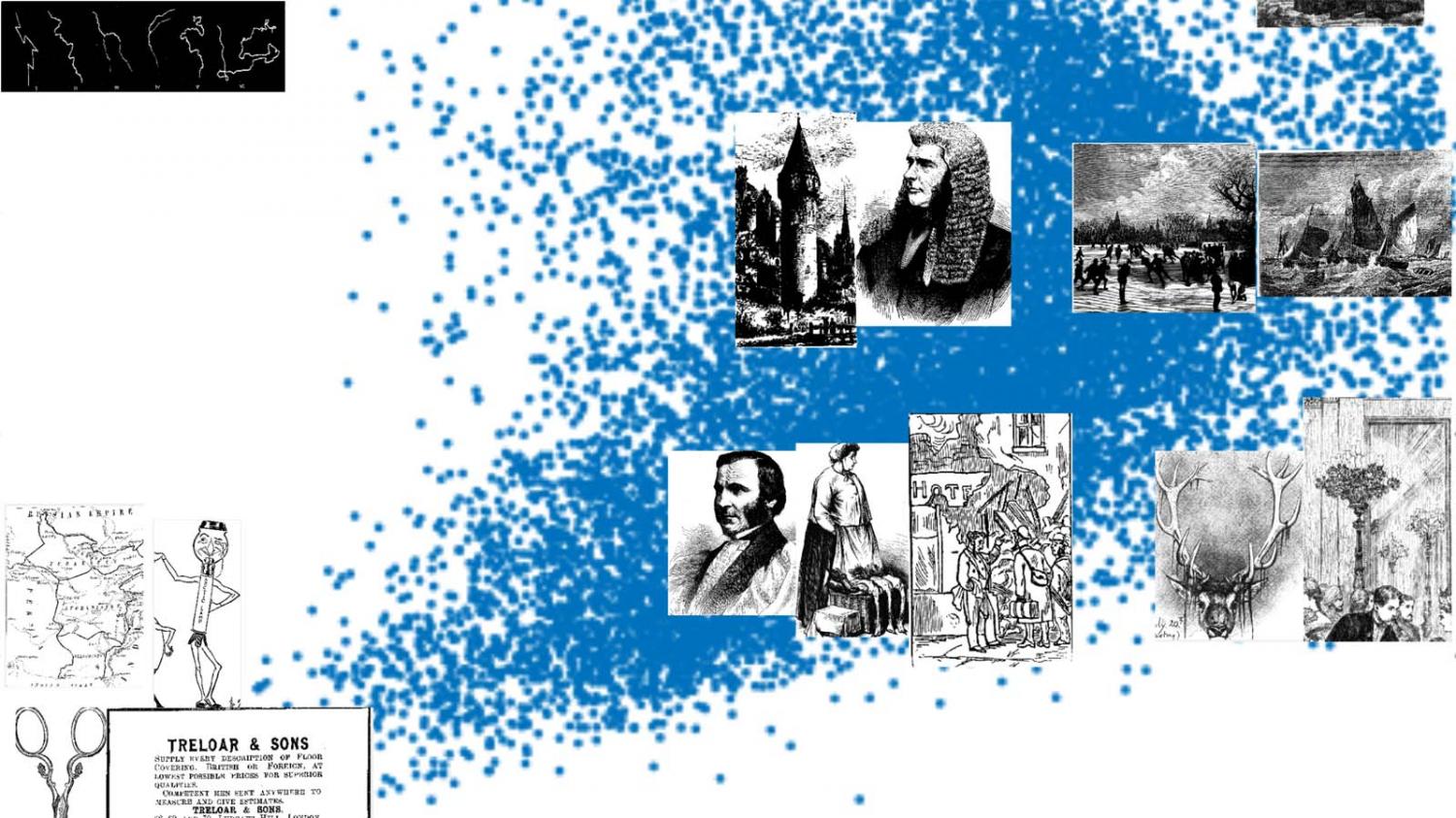
About the Project
The Illustrated Newspaper Analytics project suggests how digital humanities research on large historical data sets might move beyond text to study visual materials. Though methods of quantitative analysis, text mining, and “distant reading” have proven useful for exploring textual materials at scale, far less work has been done on computational approaches to visual materials. This results in part from technical difficulty, as historical visual materials defy many of the tools made for analyzing large media collections, including algorithms trained on photographic data. Our research pursues what methods or workflows offer the most promising approaches to large-scale computational analysis of newspaper illustrations, suggesting how researchers might adapt these techniques for a range of historical materials.
Image processing has a long history in humanities computing (Terras, Nowviskie). It has been recently revived under the category of “cultural analytics,” a phrase which urges scholars to investigate the broad range of modalities beyond text (Manovich, Piper). As digital humanities begins to explore sound, 3D cultural heritage objects, visual materials, and virtual worlds, it has come into contact with new intellectual domains. At NC State, those include the Electrical and Computer Engineering Department and the Visual Narrative cluster, which have afforded the interdisciplinary contacts to define a research program using digitized historical materials secured by the NCSU Libraries.
At the intersections of image analytics and cultural analytics, this project explores how computer vision techniques may (or may not) disclose patterns of printed images from the nineteenth century. Our data set includes runs of three illustrated periodicals which helped to pioneer the development of mass-produced illustration before halftone photography. Circulating on a large scale, Victorian illustrated periodicals inaugurated the mass image and visual communication for a general public (Anderson), whose “reading” habits have increasingly moved to multimedia experiences ever since. And yet, as Ruth Brimacombe argues, scholars have yet to sufficiently engage this visual record (191):
Arguably, one of the main reasons for the broad neglect of pictorial reportage has been the relative inaccessibility of the publications in which the images were reproduced … as well as the seemingly ephemeral nature of their original material form, the sheer number of images involved, and the lack, then and now, of a critical framework to adequately define them.
The Illustrated Newspaper Analytics project experiments with how computational techniques might help address the scale of the problem, confronting a visual archive too extensive for a human being to actually “see”. Furthermore, it proposes that computational techniques may help suggest new critical frameworks for understanding this archive. Finally, it uses the complexities of historical visual data to expose development and application challenges for computer vision.